728x90
Weight decay (commonly called L2 regularization), might be the most widely-used technique for regularizing parametric machine learning models.
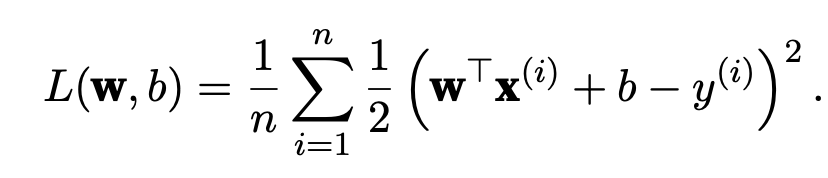

how should the model trade off the standard loss for this new additive penalty? In practice, we characterize this tradeoff via the regularization constant λ, a non- negative hyperparameter that we fit using validation data
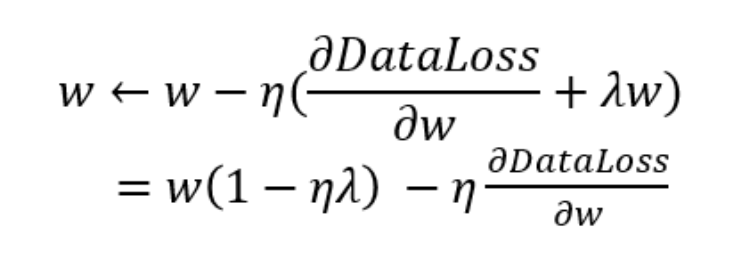
given the penalty term alone, our optimization algorithm decays the weight at each step of training.
Because weight decay is ubiquitous in neural network optimization, the deep learning framework makes it especially convenient, integrating weight decay into the optimization algorithm itself for easy use in combination with any loss function
728x90
반응형
'ComputerScience > Machine Learning' 카테고리의 다른 글
Deep Learning - 3.4 Model Selection, Underfitting, Overfitting (0) | 2022.09.27 |
---|---|
Deep Learning - 3.2~3.3 Implementation of Multilayer Perceptrons (0) | 2022.09.20 |
Deep Learning - 3.1 Multilayer perceptrons (0) | 2022.09.15 |
Deep Learning - 2.7 Concise Implementation of Softmax Regression (0) | 2022.09.01 |
Deep Learning - 2.6 Implementation of Softmax Regression from Scratch (0) | 2022.08.23 |